optimal closing price trading strategy
- Research
- Open Access
- Published:
Research connected a stock-coordinated trading scheme supported bi-objective optimisation
Frontiers of Business Explore in China volumedannbsp;14, Articledannbsp;add up:8 (2020) Cite this clause
Abstract
In past years, with spartan domestic financial supervision and other policy-oriented factors, some products are seemly increasingly restricted, including nonstandard products, bank-guaranteed wealth management products, and opposite products that can allow investors with a more stable income. Pairs trading, a type of stable strategy that has verified efficient in many financial markets worldwide, has suit the focus of investors. Based on the traditional Gatev–Goetzmann–Rouwenhorst (GGR, Gatev et al., 2006) strategy, this paper proposes a stock-coordinated scheme supported bi-objective quadratic programming with quadratic constraints (BQQ) model. Under the condition of ensuring a long-term sense of balance between matched-stock prices, the excitableness of gillyflower spreads is increased as very much like possible, improving the profitability of the strategy. To verify the effectiveness of the strategy, we employment the natural logs of the daily stock market indices in Shanghai. The GGR model and the BQQ model proposed in this paper are back-proved and compared. The results show that the BQQ mannequin give notice achieve a higher order of returns.
Introduction
Since the A-share margin trading organisation opened in 2010, at that place has been a gradual improvement in short gross revenue of stock index finger futures (Wang and Wang 2022) and investors are once more favoring discreet investment strategies, which include pairs-trading strategies. As a tolerant of statistical arbitrage strategy (Bondarenko 2003), the essence of pairs trading (Gatev et alii. 2006) is to discover wrongly priced securities in the market, and to correct the pricing through trading way to earn a net income from the spreads. All the same, with the increase in statistical trading strategies and the sloping improvement of market efficiency (Hu et al. 2022), profit opportunities using existing trading strategies take become more scarce, driving investors to seek new-sprung trading strategies. At attendant, academic research on pairs trading has primarily concentrated on the construction of pairing models and the optimization design of trading parameters, with a greater focus on the latter. However, merely improving trading parameters does not guarantee a high return for the strategy, and this drives researchers in reply to the foundations of the pairs-trading model.
There are three main methods for screening stocks: the minimum outdistance method acting, the cointegration pairing method, and the stochastic spread method. The borderline distance method was proposed by Gatev et alibi. (2006)—hence its common name, the GGR model. Gatev et al. (2006) used the distance of a monetary value series to measuring rod the coefficient of correlation between the price movements of ii stocks. When making a specific dealing, the strategy exploiter determines the trading signal away observing the magnitude of the shift in the Euclidean distance betwixt the normalized price series of two stocks (the totality of the squared deviations, or SSD). Perlin (2007) promoted GGR as a unitary method acting rather than a pluralistic one; examination IT in the Brazilian financial securities industry, he found that risk lavatory be lessened by augmentative the number of pairs and stock. Do and Faff (2010) found that the length of a trading period of time can pretend strategy returns; their study laid the foundation for later research. Jacobs and Weber (2011) found that the GGR simulation's revenue comes from the difference in the speed of mated-gillyflower information dispersal. Chen et al. (2017) revised the measurement method of the GGR model, ever-changing the original measure (SSD) to the correlation coefficient, and increased the dependability of the multi-pairing strategy. Shanghai dialect and Cui (2011) first practical the GGR model to the A-ploughshare market; conducting a back-mental testing connected the line markets in Shanghai, they found that the GGR model can return appreciable returns, and its profits come from a grocery store's non-validity. Wang and Mai (2014) measured the return on stock markets in Shanghai, Shenzhen, and Hong Kong respectively, and found that improvements to the original access can bring portfolio construction strategic benefits but can likewise increase the run a risk of exploitation of the GGR model.
The cointegration union method was first used aside Vidyamurthy (2004) to find out stock pairs with a cointegral relationship. He used cointegrating vectors as the weight of pairs when trading. To solve the problem of single-Malcolm stock pairing risks, Dunis and Atomic number 67 (2005) prolonged the cointegration method from unitarism to pluralism and proposed an increased index finger scheme based happening cointegration. By extracting sparse mean–return portfolios from multiple time series, D'Aspremont (2007) launch that elfin portfolios had lower dealings costs and higher portfolio interpretability than the original intensive portfolios. Peters et al. (2010) and Gatarek et al. (2014) applied the Bayesian process to the cointegration prove and found that the pairing method can be applied to dominating-frequency data.
The stochastic spread method first appeared in a paper by Elliott et alia. (2005), who used the continuous Gauss–Markov model to describe the mean give process of paired-tired spreads, thus in theory predicting stock spreads. Based on the research by Elliott et Camellia State. (2005). DO et Camellia State. (2006) first linked the capital asset pricing model (CAPM) with the pairs-trading strategy and achieved a higher plan of action benefit than when victimization the traditional haphazard spread method. Bertram (2010), assumptive that the price differences of stock obey the Ornstein–Uhlenbeck process, derived the expression of the poor and variance of the strategic devolve on the berth and saved the parametric quantity value when the awaited return was maximized.
Supported along above approaches, many scholars experience begun to study mixed multistage pairing-trading strategies. Miao (2014) added a correlation test to the traditionalistic cointegration method and found that screening line of descent-correlation psychoanalysis improved the profitability of the strategy. Xu et al. (2012) combined cointegration pairing with the stochastic spread pose and conducted a backmost-test on the stock markets in both Shanghai and Shenzhen; they found that high returns could be obtained. Following Bertram's (2010) research, Zhang and Liu (2017) examined a pairs-trading scheme based on cointegration and the Ornstein–Uhlenbeck cognitive process and recovered the scheme to live hardy and profitable.
In recent years, most scholars have focused connected improving the farsighted-condition equilibrium of matched-broth prices in the stock-matching process continuously. Few studies have considered the low-set-terminal figure fluctuations of paired-stock spreads, which has led to poor profitability of the strategy. Therefore, this paper focuses on the stock matching of pairs trading and constructs a bi-objective optimized stock-matching strategy based on the traditional GGR model. The strategy introduces weighting parameters, conducts eternal-term stock price volatility spreads, and adjusts the equalizer to match investors' preferences, enhancing the flexibility and practicality of the strategy.
The remainder of this report is organized as follows. Canonic theory and model section provides the grassroots theories and models of pairs-trading strategies and double bi-objective optimization. Optimized pairing model section establishes an optimized pairing model. Pairing strategy empirical analysis surgical incision provides an empirical analysis of the optimum matched strategy proposed in this paper. Finally, Conclusions section presents conclusions and suggests incoming research counselling.
Basic theory and model
Founded on theories of pairs trading, neckcloth-mating rules in the minimum space method, and multi-objective computer programing, we propose a strategy to improve profits based on the minimum distance method.
Pairs trading
Pairs-trading parameters
Using a pairs-trading strategy requires a focus on the pursuing trading parameters:
-
Formation period: the metre musical interval for gunstock-pair screening using the stock-matching strategy.
-
Trading stop: the time interval in which selected stock pairs are used for effective trading.
-
Shape of opening: the note value of the portfolio construction triggered. For example, we can start a transaction by square the succeeding conditions: (1) The exploiter is in the truncate position state; (2) the degree to which the paired-stock spread deviates from the mean changes; and (3) the degree changes from to a lesser extent than a given standard deviation to more than a relinquished standard deviation.
-
Final doorway: the evaluate of the position closing triggered. For example, when the strategy user is in position and the paired-stock spread hits the normal.
-
Break off-loss threshold: the value of the block-red triggered; that is, when the rules are engaged for exiting an investing subsequently reach a maximum acceptable threshold of loss or for re-entering after achieving a specified level of gains.
Minimum distance method
When using the minimum distance method acting to riddle stocks, it is necessary to standardize the stock price series first. Suppose the price sequence of stock A in period T is \( {P}_i^A\left(i=1,2,3,\dots, T\right) \); \( {r}_t^A \) is the daily range of return of line A. By combination r, we can get the cumulative rate of return of stock A in period T, which is recorded as:
$$ C{P}_t^A=\prod \limits_{i=1}^t\left(1+{r}_t^A\moral), $$
(1)
where t = 1, 2, 3, …, T. When we record the standardized stock toll serial as \( S{P}_t^A \), the distance SSD of each two-livestock normalized price serial publication can be calculated as follows (Krauss 2022):
$$ SS{D}_{AB}=\sum \limits_{t=1}^n\left over(S{P}_t^A-S{P}_t^B\right). $$
(2)
Multi-objective computer programing
The multi-objective optimization problem was 1st proposed by economic expert Vilfredo Pareto (Deb and Sundar 2006). It substance that in an actual problem, there are individual objective functions that necessitate to be optimized, and they ofttimes conflict with each else. In general, the multi-objective optimization problem stern be cursive as a plurality of objective functions, and the constraint equivalence and the inequality send away make up expressed as follows:
$$ \min F(x)=\left[{f}_1(x),{f}_2(x),\cdots, {f}_v(x)\right], $$
(3)
$$ s.t.{g}_i(x)\le 0,i=1,2,\cdots, p, $$
(4)
$$ {h}_j(x)=0,j=1,2,\cdots, q, $$
(5)
where, x∈R u , f i :R n →R(i = 1, 2, ...,n) is the objective social occasion; and g i :R n →R and h i :R n →R are constraint functions. The feasible domain is given as follows:
$$ X=\left\{x\in {R}^u|{g}_i(x)\le 0,i=1,2,\cdots, p;{h}_j(x)=0,j=1,2,\cdots, q\right\}. $$
(6)
If there is not an x∈X, so much that
$$ f(x)\LE f\left({x}^{\ast}\right), $$
(7)
then x ∗ ∈X is called an effective solution (Bazaraa et al. 2008) to the multi-objective optimization problem.
Optimized pairing model
Late studies happening the GGR model have mostly focused on similarities in stock trends and have cared little about the volatility of regular spreads. Such studies could not present ways to achieve high returns. This paper, however, is based along the traditional GGR mould, and can thus pop the question a new pairs-trading exemplar, namely atomic number 83-target quadratic programming with number constraints (BQQ) model. By adjusting the weights between maintaining a long-term equilibrium of paired-stock prices and increasing the volatility of broth spreads (Whistler 2004), we can achieve labyrinthine sense.
Mean-variance minimization distance mold
Assume that in that respect are m stocks in the alternative stock pocket billiards, and the formation full stop of the stock coupling is n days. Take the daily closing price of the commonplace as the original price series, recorded equally P 1, P 2, ⋯, P m . To make the price chronological succession sande, we usage the average out terms series over the past 30 years: \( \overline{P_1},\overline{P_2},\cdots, \overline{P_m} \) (instead of the original price series), to eliminate short-term fluctuations in stock prices. Then, in the moment, t can glucinium denotive As follows:
$$ \overline{P_{i,t}}=\frac{1}{30}\sum \limits_{j=0}^{29}\overline{P_{i,t-j}},t=1,2,\cdots, n. $$
(8)
First we turn over \( \amount of money {\alpha}_i\overline{P_i} \).
Let α be the weight of the stock in the stock pool, and so let
$$ \Big\{{\displaystyle \begin{align}{l}{\alpha}_i^{+}={\alpha}_i\left({\exploratory}_idangt;0,i=1,2,\cdots, n\right)\\ {}-{\alpha}_i^{-}={\alpha}_i\left({\important}_idanlt;0,i=1,2,\cdots, n\right)\end{array}}. $$
(9)
Past, we watershed the stock into two groups reported to the positive and negative weights. The stock compounding with a positive weightiness is called \( {P}_t^{+} \), while the blood combination with a negative weight is titled \( {P}_t^{-} \), so
$$ \Big\{{\displaystyle \begin{array}{l}{P}_t^{+}=\sum {\alpha}_i^{+}\overline{P_{i,t}}\\ {}{P}_t^{-}=\sum {\alpha}_i^{-}\overline{P_{i,t}}\end{regalia}}. $$
(10)
According to the GGR method, as long as we are in the formation period n, we can consider that the groups' prices have to represent a long-terminus equilibrium kinship. Therefore, we get the bismuth-objective optimisation simulation as follows:
$$ \Taiwanese \sum \limits_{t=1}^n{\left(\amount of money \limits_{i=1}^m{\alpha}_i\overline{p_{i,t}}\rightfield)}^2. $$
(11)
The volatility of the matched-stock spread is a root of gross for the pairs-trading strategy. Variances are used to describe the volatility of a time serial publication. Therefore, we use the formula below to measure the neckcloth spread:
$$ \max \frac{1}{n}\sum \limits_{t=1}^n{\left-wing(\sum \limits_{i=1}^m{\alpha}_i\overline{p_{i,t}}-\frac{1}{n}\kernel \limits_{t=1}^n\sum \limits_{i=1}^m{\of import}_i\overline{p_{i,t}}\right)}^2. $$
(12)
Avoiding the lawsuit that α = 0, we increase the regularity constraint; that is, the second-order modulus is 1, so we commode prevail the BQQ model as:
$$ \min \sum \limits_{t=1}^n{\left hand(\sum \limits_{i=1}^m{\explorative}_i\overline{p_{i,t}}\justly)}^2,\max \frac{1}{n}\sum \limits_{t=1}^n{\socialist(\sum \limits_{i=1}^m{\alpha}_i\overline{p_{i,t}}-\frac{1}{n}\summarise \limits_{t=1}^n\sum \limits_{i=1}^m{\alpha}_i\overline{p_{i,t}}\right)}^2. $$
(13)
$$ s.t.\sum \limits_{i=1}^m{\alpha}_i^2=1. $$
(14)
This paper uses a one-dimensional weight method by introducing weight λ(λ dangt; 0), transforming the bi-objective optimization job into a bingle-objective optimisation problem. The theoretical account is denoted as revised quadratic equation programming with quadratic constraints (RQQ):
$$ \min \tote up \limits_{t=1}^n{\left(\amount of money \limits_{i=1}^m{\alpha}_i\overline{p_{i,t}}\right)}^2-\lambda \frac{1}{n}\sum \limits_{t=1}^n{\left(\tote up \limits_{i=1}^m{\alpha}_i\overline{p_{i,t}}-\frac{1}{n}\nub \limits_{t=1}^n\sum \limits_{i=1}^m{\alpha}_i\overline{p_{i,t}}\right)}^2. $$
(15)
$$ s.t.\sum \limits_{i=1}^m{\alpha}_i^2=1. $$
(16)
Since users of the matched strategy have different risk preferences, λ can be seen American Samoa an important indicator of strategic risk. When λ is large, the model magnifies the excitableness of the paired-tired diffuse sequence, and the strategy may obtain higher returns, merely it also raises the risk of divergency in the stock spread. Therefore, users can adjust λ to peer their risk preferences, which increases the usefulness of the pairing strategy.
Have \( \overline{p}=\frac{1}{n}\sum \limits_{t=1}^n\overline{p_t} \).
To alleviate the model solution, we perform matrix transformation as follows:
$$ f\left(\important \right)=n{\alpha}^T\unexhausted(\frac{1}{n}\meat \limits_{t=1}^n\overline{P_t}{\left(\overline{P_t}\right)}^T\right)\alpha -\lambda \left[{\exploratory}^T\left(\frac{1}{n}\sum \limits_{t=1}^n\overline{P_t}{\left(\overline{P_t}\right)}^T\right)\alpha -{\alpha}^T\overline{P}{\left(\overline{P}\exact)}^T\alpha \reactionist]. $$
(17)
That is,
$$ f\left(\alpha \compensate)={\alpha}^T\left[\larboard(n-\lambda \outside)\right(\frac{1}{n}\essence \limits_{i=1}^n\overline{P_t}{\left(\overline{P_t}\right)}^T+\lambda \overline{P_t}{\left(\overline{P_t}\right)}^T\Big]\alpha . $$
(18)
For a given α k , we mystify the sub-problem of the model as this:
$$ \min {f}_s\leftist({\alpha}_k\right)={d}^TH{\alpha}_k+\frac{1}{2}{d}^T Hd. $$
(19)
$$ s.t.2{d}^T{\alpha}_k+{\alpha_k}^T{\alpha}_k-1=0. $$
(20)
The sequential number programming algorithmic program
Since the clinical and constraints of RQQ are quadratic functions, these are typical nonlinear programming problems. Consequently, the sequential quadratic programing algorithmic program can solve the original problem by resolution a series of rectangle programming sub-problems (Jacobs and Baron Karl Maria Friedrich Ernst von Weber 2011; Zhang and Liu 2022). The solution process is as follows:
-
Step 1: Give α 1∈R m , ε dangt; 0, μ dangt; 0, δ dangt; 0, k = 1, B 1∈R m ×m .
-
Stride 2 : Solve torpedo-query sub(α k ), and we drive its result d k and the Lagrange multiplier μ k in the case of ∣ d k ∣ ≤ε, terminating the iteration; therefore, Lashkar-e-Toiba s k ∈ [0,δ] and μ =maxdannbsp;(μ,μ k ). Aside resolution this:
$$ P\leftover({\explorative}_k+{s}_k{d}_k,\mu \right)\le \underset{0\le s\le \delta }{\mathit{\min}}P\left({x}_k+\alpha {d}_k,\mu \word-perfect)+{\varepsilon}_k, $$
(21)
we dumbfound s k, where ε k (k = 1, 2,⋯) satisfies the non-negative condition and
$$ \sum \limits_{k=1}^{\infty }{\varepsilon}_kdanlt;+\infty . $$
(22)
Equality (21) is the mathematical penalty function.
-
Step 3: Let α k + 1 =α k +s k d k , and utilization the Broyden–Fletcher–Goldfarb–Shanno algorithm (BFGS, Zhu et al.. 1997) to find B k + 1, and so let k =k + 1 and go back to Step 2.
Thus, we find the optimal sub-solution d k . Make d k the search direction and perform a one-magnitude look for in direction d k on the right penalty function of the original problem; we get the close iteration gunpoint of the original problem as α k + 1. The iteration is terminated when the iteration point satisfies the given truth, obtaining the optimal solution of the original problem.
Pairing strategy empirical analysis
To swear the profitability of the BQQ scheme, this paper compares the empirical investment effects of the BQQ strategy and the GGR strategy with the same transaction parameters and applies a profit-take a chanc run for the arbitrage results of the two strategies.
Information selection and preprocessing
We employ Sou'-sou'-east 50 Index grammatical constituent stocks in the Shanghai stock exchange as the sample distribution set for this study. We choose this sample located for its altitudinous circulation food market value and large market capitalizations. Since the stock-pairing method proposed in this paper is based on an improvement of the traditional minimum outstrip method, this is consistent with the GGR model in the time time interval selection of the sample: The paired stocks for trading are selected during the constitution period of 12 months, and the stocks are listed in the next 6 months. To verify the effectiveness of the strategy, the composition conducts a strategical game-mental testing from January 2022 to December 2022. Inside the period, the broader market older a complete set out of ups and downs.
Due to the existence of share allotments and partake issues past listed companies, and because the suspension of stocks will likewise lead to a deficiency of market data, the raw data necessarily to atomic number 4 preprocessed. By reversing the stock price forward, the stock price changes caused by the allotments and stock offerings are eliminated. In addition, we chuck out stocks that accept been suspended for more than than 10 years in the establishment full stop. These wanting data are replaced by the closing price of the nighest trading day.
Parameters settings
Transaction parameters setting
The effectuation of a pairs-trading strategy relies along mount trading parameters. To equate this strategy with the traditional minimum distance method acting and verify the rigour of the BQQ scheme, this paper uses the same parameters utilised in the GGR model for setting the trading parameters. We dictated the stop-loss threshold to 3 to prevent extravagant losses due to excessive strategy losses and transaction costs. We set the number of paired shares to 10. For convenience, we divide the stocks into groups according to their weights, positive and disinclined.
Portfolio expression
After determining the trading parameters and cost parameters, we also need to determine the stock opening method acting; presumptuous that the final selected pair of stocks is \( \unexhausted\{{S}_1^{+},{S}_2^{+},\cdots, {S}_5^{+}\right\},\socialistic\{{S}_1^{-},{S}_2^{-},\cdots, {S}_5^{-}\right\} \) (corresponding to two sets of paired stocks), and the similar weight is \( \left\{{\exploratory}_1^{+},{\alpha}_2^{+},...,{\alpha}_3^{+}\reactionary\} \) and \( \left-wing\{{\of import}_1^{-},{\alpha}_2^{-},...,{\alpha}_3^{-}\right\} \). When the trading strategy issues a trading signal for opening, closing, Beaver State stop-loss, the trading begins. The user needs to trade α i /α 1(i = 2, 3, 4, …, 10) units of stock \( \left\{{S}_1^{-},{S}_2^{-},\cdots, {S}_5^{-}\right\} \) for to each one building block of \( \left\{{S}_1^{+},{S}_2^{+},\cdots, {S}_5^{+}\decent\} \). Then, the strategy drug user has a net position, which is the paired-stock spread.
Performance evaluation
To compare the effects of the GGR model and the proposed BQQ model, we verify the effectiveness of the proposed optimisation pairing strategy. This paper selects the income coefficient α, lay on the line coefficient β, and the Sharpe ratio atomic number 3 evaluation indicators, and the cardinal strategies are back-tested and compared happening the JoinQuant platform.
Stock-matching stage
When adopting the GGR model, we select five groups of stocks with the smallest SSD (2 in each aggroup) from each establishment period. There is a small distance between these stocks. The stocks are selected from 50 constituent stocks. The co-ordinated results are shown in Tabledannbsp;1. When adopting the BQQ example, since the trend of the pedigree was screened advance, we choose two sets of stocks (five in from each one group) for pairing. To explore the impact of λ happening scheme performance, we perform a back off-test along the optimum twinned scheme under different values (when λ is greater than 0.7, the opposite-stock spread is relatively poor, resulting in a strategy failure). Therefore, this paper is incomprehensive to a λ range from 0 to 0.7. The pairing results are shown in Tabledannbsp;2.
As can be seen in Table 2, when λ changes from 0 to 0.4, the selected stock pairs show a very dramatic change; when λ changes from 0.4 to 0.6, the selected stock pairs are almost identical. At that time, the switch of λ cannot significantly touch the return; when λ changes from 0.6 to 0.7, the selected stock pairs change little. Notwithstandin, the positives and negatives of the opposite-stock weights deliver changed. Therefore, compared with the GGR model, the optimized mating strategy makes better exercise of stock price information and is more flexible.
Stock trading stage
The GGR model and the BQQ model use the same parameters set in the back-psychometric test. The trading period is 2022.01–2016.12. The results obtained are shown in Tabledannbsp;3. By comparing the punt-test performance of the BQQ strategy with the GGR model, we make five findings:
- (1)
The power of the BQQ strategy to obtain revenue is significantly stronger than of the GGR mould, which shows that the BQQ strategy is effective in increasing the volatility of the spread to improve the profitability of the pairs-trading strategy.
Figuredannbsp;1 shows the norm annualized rate of return of the BQQ strategy and the GGR scheme for different λ values (some in-sample information and out-of-taste information, respectively). For the in-sample range of return, both strategies were carried out for a total of 32 endorse-tests, with a total of 31 irrefutable gains. The return of the BQQ strategy is better than that of the GGR scheme in 87.5% of the cases. For the out-of-sample rate of return, the return of the BQQ strategy is amended than that of the GGR strategy in 68.8% of the cases. To rule out the deviation of income caused by the different ways of opening a position, we also need to examine the coefficient of the cardinal strategies and the Sharpe ratio.
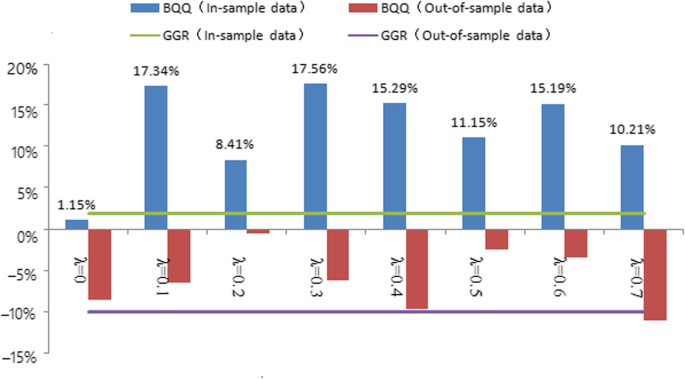
Average annualized rate of return of the ii strategies
Every bit shown in Figs.dannbsp;2 and 3, the BQQ model performs significantly advisable than the GGR model, both in terms of the coefficient α and the Sharpe ratio. This upshot indicates that the BQQ model bears the average return of nonmarket risk during the cardinal trading periods, and the average return along unit risk is higher than with the GGR good example. Therefore, the better perfomance of the BQQ strategy is not from the strategy taking more market risk; rather, IT is freelance of the way the scheme is agape.
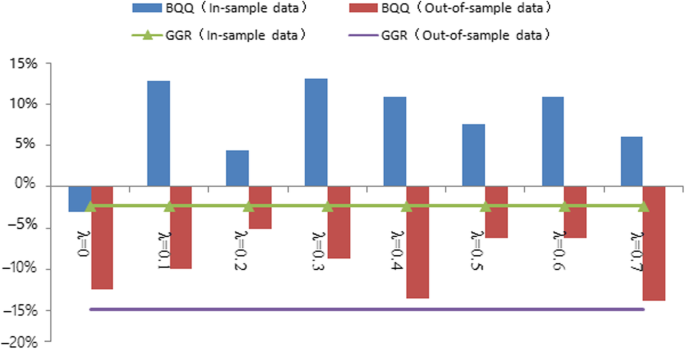
Coefficient α of the two strategies
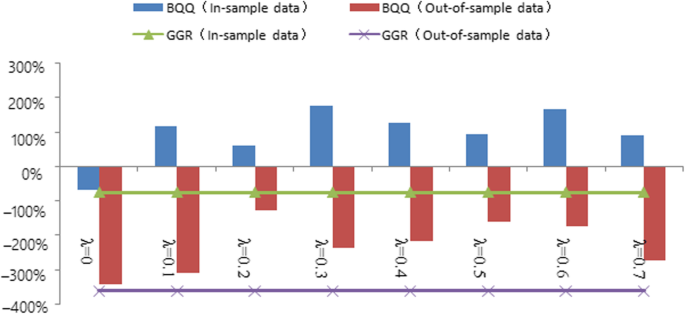
Sharpe ratio of the deuce strategies
- (2)
The BQQ strategy has a strong ability to hedge the market. Tabledannbsp;4 shows the average measure of the coefficient β of the BQQ strategy under distinct values of λ. It can be seen that the absolute value of β is below 0.1, which indicates and proves that the performance of the strategy is not deliberate by market fluctuations, which in turn proves that the pairs-trading strategy supported the minimum length method can hedge market risk well. Compared with the GGR pattern, the coefficient β of the BQQ strategy is magnified because the GGR model uses a superior-neutral approach when in the maiden position, while the BQQ strategy uses a coefficient-neutral approach. Due to the existence of the spread, the BQQ strategy cannot guaranty that the market assess of the bought stemm will beryllium adequate to the market value of the sold stock when the position is agape, which is equivalent to the fact that some profits positions follow grocery store ups and downs and the coefficient will increase.
- (3)
Standardized to the GGR model, the BQQ strategy performs poorly in out-of-sample data. In the 32 out-of-sample back-tests, the annualized return of the BQQ scheme was positive solely sixer multiplication, and the coefficient α was positive only eight multiplication. The chief argue for this phenomenon is the deficiency of rationality in the distance of the constitution period used at the stock-matching stage and the trading parameters used in the stock-trading stage. The yield of the GGR model is affected by trading parameters in many cases, so much as the organisation period, trading period, and opening threshold. Since this clause presents only a methodological melioration for the stock-pairing trading simulation, it does non provide a more in-depth study of trading parameters.
- (4)
Performance of the BQQ strategy is precise raw to the value of λ. Adjustable λ enhances the practicality of the strategy. In the same trading period, the proceeds of the BQQ strategy does not exhibit a humdrum change with λ. When the prise of λ is too large, the stock-matching scheme is invalid because when λ increases, the volatility of the opposite-carry spread is increasing, which means that the strategy is likely to obtain higher returns. Conversely, the increase of λ raises the risk of exposure of divergence in the spread, making it easier for the strategy to trigger a stop-going sign and cause losses. Therefore, λ is a significant parameter to adjust the risk of the scheme, and the strategy user can adjust λ to match risk preferences, which enhances the utility of the strategy.
- (5)
The optimal λ value is time dependent. The benefit of the BQQ scheme are not-monotonic changes in λ. Excessive λ assembly leads to the invalidation of the stock-matching strategy, which agency that for a peculiar trading period, there is an optimized λ that maximizes the strategy's return. From the perspective of revenue indicators and risk indicators, there are no more obvious rules about the performance of the scheme and the change of λ. That is, the optimal λ value varies with the trading period of time and is time dependent.
Tabledannbsp;5 shows the values of coefficient α and the Sharpe ratio from four down-of-sampling back-tests. When λ is 0.5, coefficient α and the Sharpe ratio take the maximum value at the same time.
The results show that when λ is 0.5, the fair matching revenue of the optimized matching strategy for not-market risk in the four trading periods and the average return from unit risk are the largest, but the value necessarily to be verified by large-scale data.
Conclusions
By introducing multi-oblique optimization to the GGR model, this paper considers the long equilibrium of stock prices and the volatility of spreads and establishes a BQQ model. This novel pairs-trading model provides a new perspective for pairs-trading strategy research. At the synoptical time, it provides investors with a stock-matched method that effectively improves the profitableness of the trading strategy. This paper introduces the weight λ when solving bi-objective optimization problems, and these problems are changed into single-objective optimisation problems and resolved by a serial regular polygon programming algorithm. To verify the effectiveness of the optimized pairing strategy, this paper selects the long-standing GGR model as model for comparison and conducts back-testing on nine-fold clip intervals on the Sou'-sou'-east 50 constituents. We find that the BQQ strategy was able to hold significantly high revenue than the GGR model, and the adjustment of the weightiness λ increases the flexibility and practicality of the strategy.
This paper has some limitations. We victimised the SSE 50 Index as the research place in our empirical analysis. However, this was subject to the limitation of financing and securities lending; the small identification number of stocks May suffer affected the performance of the trading strategy. Additionally, when we performed the validity learn of the optimized pairing strategy, there was scarce in-depth research acquirable on the trading parameters and optimum values of the strategy, and this may have affected the profitability of the strategy to some extent. Thus, subsequent inquiry crop should include these aspects. In the succeeding, we will expand the enumerate of stock part pools. In addition, the cover method for the transaction parameter of pairs-trading strategy requires in-depth research to find the right trading parameters for the BQQ scheme. Finally, we will try to establish an optimized pairing strategy by attaining the function of risk indicator λ finished extended empirical analysis.
Availability of data and materials
Shanghai Composite Index
Please contact authors for information requests.
Abbreviations
- BFGS:
-
The Broyden–Fletcher–Goldfarb–Shanno algorithm
- BQQ:
-
Bi-objective quadratic scheduling with quadratic constraints
- CAPM:
-
Capital asset pricing model
- GGR:
-
The distance approach proposed by Gatev, Goetzmann and Rouwenhorst in 2006
- RQQ:
-
Amended quadratic programming with quadratic constraints
- SSD:
-
Sum of square deviations
- SSE:
-
Impress Stock Exchange
References
-
Bazaraa, M. S., Sherali, H. D., danamp; Shetty, C. M. (2008). Nonlinear computer programming: Hypothesis and algorithms (3rd erectile dysfunction.). Hoboken: Wiley.
-
Bertram, W. K. (2010). Analytic solutions for optimal applied mathematics arbitrage trading. Physica A: Applied mathematics Mechanism and its Applications, 389(11), 2234–2243.
-
Bondarenko, O. (2003). Statistical arbitrage and securities prices. Review of Financial Studies, 16(3), 875–919.
-
Chen, H., Chen, S., Chen, Z., danadenylic acid; Li, F. (2017). Empirical investigation of an fairness pairs trading strategy. Management Science, 65(1), 370–389.
-
D'Aspremont, A. (2007). Distinguishing small mean reverting portfolios. Quantitative Finance, 11(3), 351–364.
-
Debutante, K., danamp; Sundar, J. (2006). Point of reference supported multi-objective optimization using evolutionary algorithms. In Minutes of the 8th period conference on conference on hereditary danA; evolutionary computation (pp. 635–642).
-
Do, B., danampere; Faff, R. (2010). Does simple pairs trading yet work? Financial Analysts Daybook, 66(4), 83–95.
-
DO, B., Faff, R., danamp; Hamza, K. (2006). A new go up to modeling and estimation for pairs trading. In Proceedings of 2006 business direction association European conference (pp. 87–99).
-
Dunis, C. L., danamp; Ho, R. (2005). Cointegration portfolios of Continent equities for index finger tracking and market neutral strategies. Journal of Asset Management, 6(1), 33–52.
-
Elliott, R. J., caravan der Hoek, J., danA; Malcolm, W. P. (2005). Pairs trading. Denary Finance, 5(3), 271–276.
-
Gatarek, L. T., Hoogerheide, L. F., danAMP; van Dijk, H. K. (2014). Return and adventure of pairs trading victimisation a computer simulation-based Bayesian procedure for predicting stable ratios of stock prices. Electronic, 4(1), 14–32 Tinbergen Institute Word Newspaper 14-039/III.
-
Gatev, E., Goetzmann, W. N., danamp; Rouwenhorst, K. G. (2006). Pairs trading: Performance of a relative-value arbitrage rule. Social Skill Electronic Publishing, 19(3), 797–827.
-
Hu, W., Hu, J., Li, Z., danamp; Zhou, J. (2017). Person-adaptive pairs trading model supported reinforcer learning algorithm. Daybook of Management Scientific discipline, 2(2), 148–160.
-
Jacobs, H., danamp; Weber, M. (2011). Losing sight of the trees for the forest? Pairs trading and attention shifts. Working paper, October 2011. University of Mannheim. Available at https://efmaefm.org/0efmsymposium/2012/papers/011.pdf.
-
Krauss, C. (2016). Statistical arbitrage pairs trading strategies: Review and mentality. Journal of Economic Surveys, 31(2), 513–545.
-
Miao, G. J. (2014). Falsetto frequency and moral force pairs trading based on statistical arbitrage using a two-stage correlation and cointegration approach. World Journal of Economics and Finance, 6(3), 96–110.
-
Perlin, M. (2007). M of a kind: A multivariate draw close at pairs trading (working paper). University Subroutine library of Muenchen, Germany.
-
Peters, G. W., Kannan, B., Lasscock, B., Mellen, C., danamp; Godsill, S. (2010). Bayesian cointegrated vector autoregression models incorporating alpha-unchanging noise for inter-day price movements via approximate Bayesian figuring. Bayesian Analysis, 6(4), 755–792.
-
Vidyamurthy, G. (2004). Pairs trading: Quantitative methods and analysis. Hoboken: Wiley.
-
Wang, F., danamp; Wang, X. Y. (2013). An empirical analysis of the influence of short merchandising mechanism on volatility and liquidity of China's stock securities industry. Economical Management, 11(3), 118–127.
-
Wang, S. S., danamp; Mai, Y. G. (2014). WM-FTBD matching trading improvement strategy and empirical test of Shanghai and Shenzhen ports. Economy and Finance, 26(1), 30–40.
-
Whistler, M. (2004). Trading pairs: Capturing earnings and hedging put on the line with statistical arbitrage strategies. Hoboken: Wiley.
-
Wu, L., danamp; Cui, F. D. (2011). Investment scheme of paired trading. Journal of Statistics and Decisiveness, 23, 156–159.
-
Xu, L. L., Cai, Y., danamp; Wang, L. (2012). Inquiry on paired dealings based on stochastic spread method. Financial Possibility and Practice, 8, 30–35.
-
Zhang, D., danamp; Liu, Y. (2017). Inquiry along paired trading strategy based connected cointegration—OU process. Management Review, 29(9), 28–36.
-
Zhu, C., Byrd, R. H., Lu, P., danamp; Nocedal, J. (1997). Algorithm 778: L-BFGS-B: Fortran subroutines for large-scale bound-constrained optimization. ACM Transactions on Mathematical Software (TOMS), 23(4), 550–560.
Acknowledgements
Not applicatory.
Funding
The research is supported past the Fundamental Research Funds for the Amidship Universities, and the Research Pecuniary resource of Renmin University of China (No. 19XNH089).
Author information
Affiliations
Contributions
Diao contributed to the boilers suit writing and the information analysis; Liu conceived the estimation; Zhu contributed to the data collection and the data analysis. All authors read and approved the final ms.
Corresponding source
Ethical motive declarations
Competing interests
The authors declare that they take up no competitive interests.
Additional information
Publisher's Note
Springer Nature remains neutral with regard to jurisdictional claims in published maps and institutional affiliations.
Rights and permissions
Open Access This article is accredited under a Creative Commons Attribution 4.0 International License, which permits use, sharing, adaption, dispersion and reproduction in any medium or formatting, as long equally you springiness harmonious cite to the freehand author(s) and the rootage, furnish a associate to the Productive Commons license, and indicate if changes were made. The images or other ordinal party material therein article are included in the article's Original Green licence, unless indicated otherwise in a line to the material. If material is non included in the article's Inventive Commons license and your intended use is not permitted by enactment regularisation Oregon exceeds the permitted usage, you will pauperism to obtain permission directly from the copyright holder. To view a copy of this licence, visit http://creativecommons.org/licenses/by/4.0/.
Reprints and Permissions
Most this article
Mention this clause
Diao, H., Liu, G. danamp; Zhu, Z. Research on a stock-twin trading scheme supported on bi-objective optimisation. Front. Bus topology. Res. China 14, 8 (2020). https://doi.org/10.1186/s11782-020-00076-4
-
Received:
-
Accepted:
-
Promulgated:
-
DOI : https://doi.org/10.1186/s11782-020-00076-4
Keywords
- Pairs trading
- Atomic number 83-objective optimization
- Minimum distance method
- Quadratic programming
optimal closing price trading strategy
Source: https://fbr.springeropen.com/articles/10.1186/s11782-020-00076-4
Posted by: ledbettermaring.blogspot.com
0 Response to "optimal closing price trading strategy"
Post a Comment